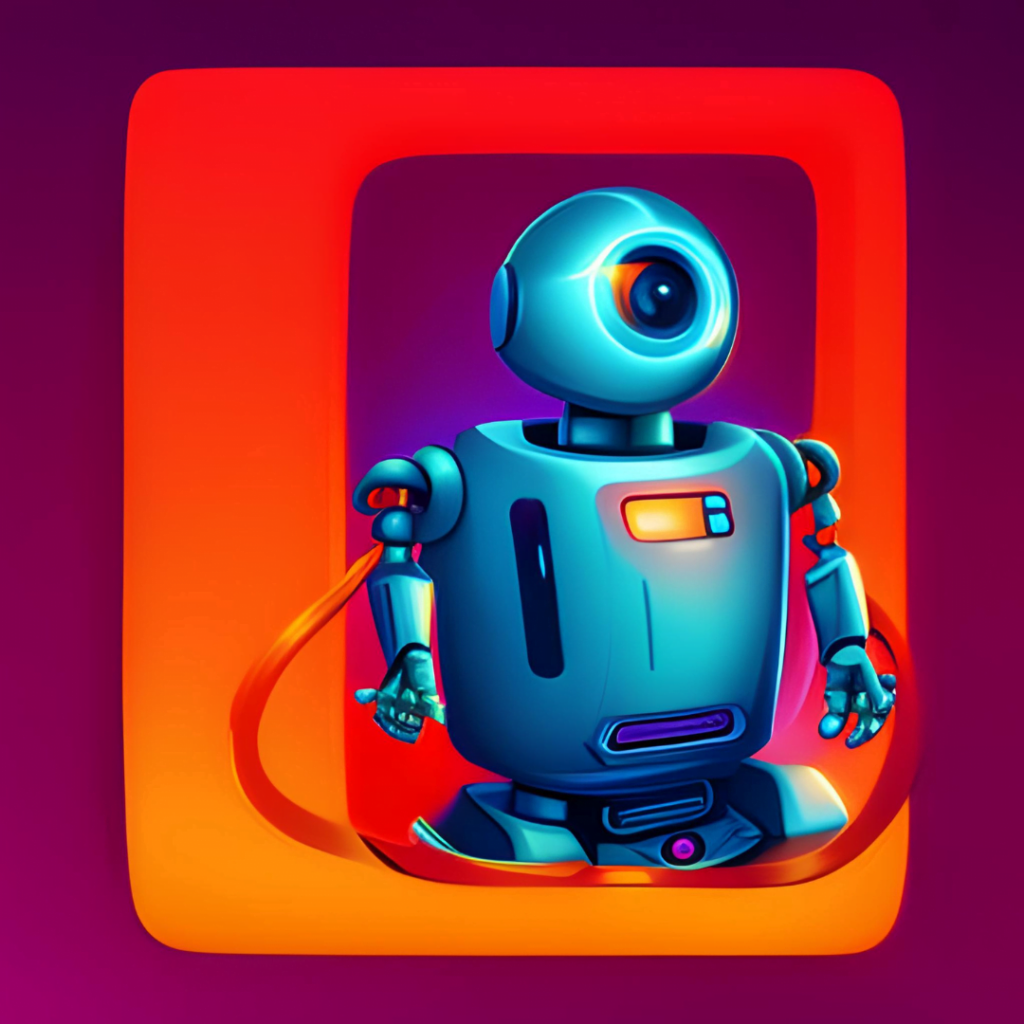
Machine Learning has been one of the most discussed topics in the world of technology in recent years. It is a subset of Artificial Intelligence (AI) that allows machines to learn and improve their performance without being explicitly programmed.
Machine Learning involves the use of algorithms that can learn from data and make predictions or decisions based on that learning. In this article, we will explore what Machine Learning is, how it works, what it is used for, and some examples of it in action.
What is Machine Learning?
At its core, Machine Learning is a technique that enables machines to learn from data and improve their performance on a specific task. It is based on the idea that machines can automatically learn patterns from data, identify hidden relationships, and make predictions or decisions based on that learning.
Related article: What is Artificial Intelligence (AI)? AI Explained Simply
In other words, Machine Learning algorithms are designed to improve their performance over time by learning from the data they are given.
How Does Machine Learning Work?
Machine Learning works by using algorithms that are designed to learn from data. These algorithms are fed data in the form of inputs, which can be anything from text, images, or even sound. The algorithms then analyze the data and identify patterns or relationships within it. Based on this analysis, the algorithms make predictions or decisions about the data.
There are three main types of Machine Learning: supervised learning, unsupervised learning, and reinforcement learning.
1. Supervised learning
Supervised learning is the most common type of Machine Learning. It involves training a model on labeled data, which means that the data is already categorized. In supervised learning, the algorithm is provided with inputs and corresponding correct outputs, called labels. The algorithm then learns to map the inputs to the correct outputs by adjusting the parameters of the model. Once the model has been trained, it can be used to make predictions on new, unseen data.
Supervised learning is often used for tasks like classification and regression. Classification involves predicting a discrete output, such as whether an email is spam or not. Regression involves predicting a continuous output, such as the price of a house based on its features.
2. Unsupervised learning
Unsupervised learning involves training a model on unlabeled data, which means that the algorithm must find patterns or relationships on its own. In unsupervised learning, the algorithm is provided with inputs but not corresponding labels. The algorithm then learns to identify patterns or relationships in the data without any guidance.
Unsupervised learning is often used for tasks like clustering and dimensionality reduction. Clustering involves grouping similar data points together based on their characteristics. Dimensionality reduction involves reducing the number of variables in a dataset without losing important information.
3. Reinforcement learning
Reinforcement learning involves training a model to make decisions based on rewards and punishments. In reinforcement learning, the algorithm learns to take actions that maximize a reward signal. The algorithm receives feedback in the form of a reward signal based on its actions, and it learns to adjust its behavior to maximize the reward.
Aging from 3 to 100 years old in 14 seconds using Runway & Midjourney 😱 @runwayml @midjourney #midjourney #runway #AI
— AI Trendz (@AiTrendz) March 20, 2023
Credit: Christen Ortner pic.twitter.com/6tJhENe4bJ
Reinforcement learning is often used for tasks like game playing and robotics. In game playing, the algorithm learns to take actions that lead to winning the game. In robotics, the algorithm learns to take actions that lead to completing a task, such as navigating a maze or picking up an object.
What is Machine Learning Used for?
Machine Learning has many practical applications across various industries. In healthcare, it is used for predicting diseases and identifying the best treatment options. In finance, it is used for fraud detection and stock market analysis.
In marketing, it is used for customer segmentation and targeted advertising. In manufacturing, it is used for predictive maintenance and quality control. In transportation, it is used for route optimization and autonomous vehicles.
What is Machine Learning vs AI?
Machine Learning is a subset of AI, which means that it is a specific application of AI. AI refers to any system or technology that can perform tasks that would normally require human intelligence, such as speech recognition, image recognition, and decision-making.
AI encompasses a wide range of techniques and approaches, including Machine Learning, as well as other techniques such as rule-based systems, genetic algorithms, and expert systems. It involves the use of algorithms that can learn from data and make predictions or decisions based on that learning.
Machine Learning algorithms are designed to improve their performance over time by learning from the data they are given. The goal of Machine Learning is to create models that can generalize from examples and make accurate predictions or decisions on new data.
🔷 @BillGates has recently wrote an article about the arrival of AI and his excitement for it.
— AI Trendz (@AiTrendz) March 28, 2023
"I knew I had just seen the most important advance in technology since the graphical user interface."https://t.co/IuVrPyNXQn#billgates #AI #ArtificialIntelligence
One way to think about the difference between AI and Machine Learning is that AI is the broader field that encompasses many different approaches, while Machine Learning is a specific technique within that field that uses statistical methods to learn from data. Another way to think about it is that AI is the goal, while Machine Learning is one of the tools used to achieve that goal.
Another key difference between AI and Machine Learning is that AI is often focused on creating systems that can perform tasks that would normally require human intelligence, while Machine Learning is focused on creating models that can make accurate predictions or decisions based on data. For example, an AI system might be designed to recognize speech, while a Machine Learning model might be trained to predict which customers are most likely to churn.
What is Machine Learning with Examples?
There are many examples of Machine Learning in action today. One popular example is the recommendation systems used by companies like Amazon and Netflix. These systems use Machine Learning algorithms to analyze user data and recommend products or movies based on that analysis.
Another example is image recognition, which is used in applications like facial recognition, security systems, and self-driving cars. In healthcare, Machine Learning is used for predicting diseases and identifying the best treatment options.
Unpopular Facts about Machine Learning:
- Despite being a powerful tool, Machine Learning models are not always accurate, and their predictions can be biased or flawed.
- Machine Learning algorithms can be fooled by adversarial attacks, where a malicious actor manipulates the input data to trick the algorithm into making the wrong decision.
- Machine Learning requires large amounts of data to be effective, which can raise privacy concerns and ethical issues.
- The development of Machine Learning models requires significant computing power and resources, which can be a barrier to entry for smaller organizations.
- Machine Learning models can become outdated quickly as new data becomes available, which requires constant monitoring and updating.
Bottom line
Machine Learning is a powerful technology that has the potential to revolutionize many industries. It is a subset of Artificial Intelligence that allows machines to learn and improve their performance without being explicitly programmed. Machine Learning algorithms can learn from data and make predictions or decisions based on that learning.
While Machine Learning has many advantages, it also has some challenges. Machine Learning models are not always accurate, and their predictions can be biased or flawed. Adversarial attacks can manipulate the input data to trick the algorithm into making the wrong decision. Machine Learning requires large amounts of data to be effective (like in the case of ChatGPT), which can raise privacy concerns and ethical issues.
The development of Machine Learning models requires significant computing power and resources, which can be a barrier to entry for smaller organizations. Finally, Machine Learning models can become outdated quickly as new data becomes available, which requires constant monitoring and updating.
In conclusion, Machine Learning is a rapidly evolving field with many practical applications. It has the potential to revolutionize many industries, but it also has some challenges that must be addressed. As the technology continues to advance, it will be important to consider the ethical implications and ensure that Machine Learning is used responsibly.