Imagine AI that can think, reason, and learn like humans. That's Artificial General Intelligence (AGI), and it could revolutionize our world!

Artificial Intelligence (AI) is having a transformative impact on our society. Ever since the inception of AI, researchers and developers have been trying to create machines that can think and reason like humans.
The ultimate goal of AI is to create Artificial General Intelligence (AGI). AGI is a machine that can perform any intellectual task that a human can (but without creativity). This article will explore what AGI is, how it works, and its potential applications.
Understanding Artificial General Intelligence (AGI)
Artificial General Intelligence (AGI) is the next step in AI. AGI represents a machine that can think and reason like a human, understands language, and can perform any intellectual task that a human can. AGI is considered to be the holy grail of AI.
Related article: TOP 10 AI startups in 2023 (part 1)
AGI is a machine that has the cognitive abilities of a human being. Such a machine would be capable of logical reasoning, abstract thinking, and problem-solving. AGI would be able to learn and adapt to new situations, make decisions, understand language, and have a general perception of the world like a human being.
Defining Artificial General Intelligence
Artificial General Intelligence can be defined as a machine that has the cognitive abilities of a human being. It is an AI system that can perform any intellectual task that a human can. Such a machine would be capable of logical reasoning, abstract thinking, and creative problem-solving.
AGI would be able to learn and adapt to new situations, make decisions, understand language, and have a general perception of the world like a human being. AGI is the ultimate goal of AI research, and many experts believe that it will be achieved in the coming years. The development of AGI is a major challenge for AI researchers.
Artificial General Intelligence (AGI) might be closer than you think! 🙆♂️#ai #agi #robot #robotics #ml pic.twitter.com/sDhT64EJy9
— AI Trendz (@AiTrendz) April 8, 2023
The machine must be able to perform tasks that require human-like intelligence, such as understanding natural language, recognizing objects and patterns, and making decisions based on incomplete or ambiguous information. Achieving AGI requires a combination of advanced algorithms, powerful computing resources, and massive amounts of data.
Comparing AGI to Narrow AI
Most of today’s AI systems are designed for specific tasks and are called narrow AI. These systems are designed to perform a specific task and are not versatile. For example, a self-driving car can only drive itself, and a virtual assistant can only answer specific questions. Narrow AI systems are designed to perform a specific task and are not capable of performing any other task.
On the other hand, AGI is an artificial intelligence system that can perform any intellectual task that a human being can, while a narrow AI system can only perform specific tasks. While narrow AI systems are useful for specific tasks, they are not capable of performing tasks that require human-like intelligence.
For example, a virtual assistant can answer specific questions, but it cannot understand the context of the question or provide a nuanced response. AGI, on the other hand, would be able to understand the context of the question and provide a nuanced response.
Key Characteristics of AGI
The key characteristics of AGI include reasoning, problem-solving, learning, perception, and natural language understanding. These are the cognitive abilities of a human being, and an AGI machine would have these abilities. AGI would be able to reason and solve complex problems, learn from experience, perceive the world like a human being, and understand natural language.
Read more: 8 Useful AI Prompts to Master ChatGPT (With Examples)
AGI would be able to understand the context of a conversation, recognize emotions, and respond appropriately. This would enable AGI to interact with humans in a more natural way. AGI would also be able to learn from experience and adapt to new situations, making it more versatile than narrow AI systems.
The Evolution of Artificial Intelligence
The journey to AGI has been a long one. It started back in the 1950s when the first AI program was written. Since then, there have been significant developments in the field of AI. However, the path to AGI is still a challenging one.
A Brief History of AI Development
The first AI program called the Logic Theorist was written by Allen Newell and J.C. Shaw. The program was able to prove mathematical theorems. In 1956, John McCarthy organized the Dartmouth Conference, which is considered the birthplace of AI. The conference brought together researchers who were interested in exploring the potential of AI.

In the 1960s, researchers focused on symbolic AI, which uses logic-based approaches to solve problems. However, this approach had limitations, and researchers started exploring other methods. In the 1980s and 1990s, neural networks and machine learning became popular. These approaches allowed machines to learn from data and make predictions.
Today, researchers are exploring different approaches, such as deep learning, reinforcement learning, and hybrid models. Deep learning involves training neural networks on large datasets, while reinforcement learning involves training machines to make decisions based on rewards and punishments. Hybrid models combine different approaches to achieve better performance.
Milestones in AI Research
There have been several milestones in AI research. One of the earliest milestones was the development of expert systems. These systems were designed to mimic the decision-making ability of human experts in specific domains. Another milestone was the development of natural language processing, which allowed machines to understand and generate human language.
This is #AI-driven garbage-sorting robot!😍
— AI Trendz (@AiTrendz) April 24, 2023
The AMP Cortex system sorts photographs of recyclables into hundreds of categories based on material type, color, shape, & other features of the objects captured in the images📷
The future is AI! @AMPRobotics pic.twitter.com/Xi1XRoNewy
In recent years, AI has made significant progress in speech recognition, machine translation, and game-playing. For example, Google’s AlphaGo program was able to defeat the world champion in the game of Go. However, AGI remains an elusive goal.
The Road to AGI: Current State of AI
The current state of AI is impressive. Researchers are making progress in different areas of AI, and there have been significant advances in natural language processing, image recognition, and robotics. For example, self-driving cars are becoming a reality, and robots are being used in manufacturing and healthcare.
However, AGI requires a machine that can reason like a human being and learn from its experiences. This is still a long way off. One of the challenges is developing machines that can learn from a small amount of data, like humans can. Another challenge is developing machines that can understand context and make decisions based on it.
Despite these challenges, researchers are optimistic about the future of AI. They believe that AGI is achievable, although it may take several decades to achieve. In the meantime, AI will continue to transform industries and improve our lives in countless ways.
Approaches to Developing AGI
Artificial General Intelligence (AGI) is an area of research that aims to develop intelligent machines that can perform tasks that require human-level intelligence. Researchers are exploring different approaches to developing AGI. Some of these approaches include symbolic AI, neural networks, and reinforcement learning.
Symbolic AI and Knowledge Representation
Symbolic AI uses logic-based approaches to solve problems. It represents knowledge in terms of symbols and performs logical operations on them. For example, a symbolic AI system can represent the rules of a game and use logical inference to make decisions.
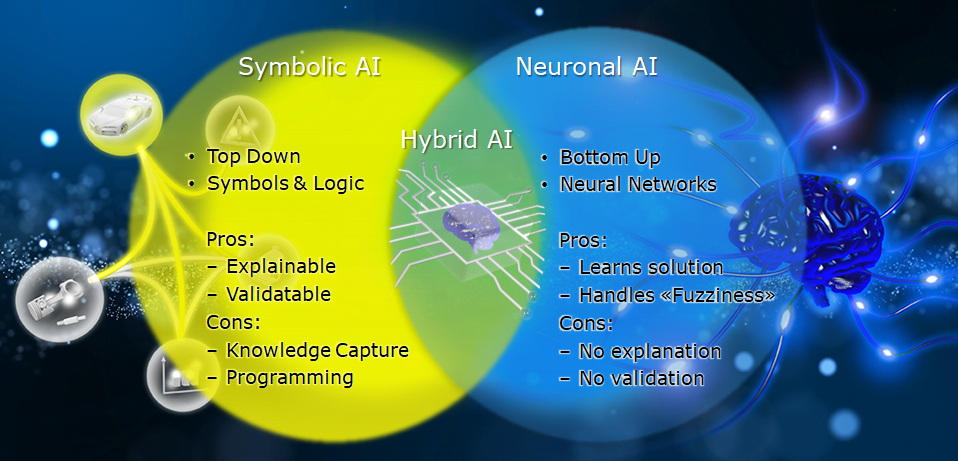
While symbolic AI has been successful in specific areas, it has limitations when it comes to dealing with uncertainty and ambiguity. One of the challenges in symbolic AI is knowledge representation. Representing knowledge in a way that is both accurate and efficient is a difficult task.
For instance, representing the knowledge required to understand a natural language sentence can be challenging. Researchers are exploring different approaches to knowledge representation, such as ontologies and semantic networks.
Neural Networks and Deep Learning
Neural networks and deep learning have made significant progress in recent years. These approaches learn from large amounts of data and can recognize patterns in data. However, their ability to reason and understand language is still limited.
One of the challenges in neural networks is overfitting. Overfitting occurs when a model is too complex and fits the training data too closely, resulting in poor generalization to new data. Researchers are exploring different techniques to prevent overfitting, such as regularization and early stopping.
Reinforcement Learning and Decision Making
Reinforcement learning is an approach where an agent learns to make decisions in a dynamic environment. It is based on the concept of rewards and penalties. The agent learns from its experiences and tries to maximize its rewards. Reinforcement learning has been successful in game-playing and robotics.
Also read: TOP 10 AI startups in 2023 You Need to Watch (part 2)
One of the challenges in reinforcement learning is exploration-exploitation trade-off. Exploration is the process of trying out new actions to learn about the environment, while exploitation is the process of using the current knowledge to maximize rewards. Finding the right balance between exploration and exploitation is a difficult task.
Hybrid Approaches and Integrated Systems
Hybrid approaches and integrated systems combine different approaches to create more powerful AI systems. For example, a system that combines symbolic AI with neural networks can reason and learn from data. Such systems can be used in robotics, natural language processing, and image recognition.
One of the challenges in hybrid approaches is integration. Integrating different approaches can be difficult due to differences in representation, learning, and reasoning. Researchers are exploring different techniques to integrate different approaches, such as knowledge-based learning and transfer learning.
Potential Applications of AGI
There are several potential applications of AGI, ranging from transforming industries to healthcare and medicine.
Transforming Industries with AGI
AGI has the potential to transform industries such as finance, manufacturing, and transportation. An AGI machine can make predictions, identify patterns, and optimize processes. This can lead to increased productivity, cost savings, and efficiency.
1. AGI in Healthcare and Medicine
AGI can play a significant role in healthcare and medicine. For example, an AGI machine can analyze medical data, make diagnoses, and develop treatment plans. AGI can also be used in drug discovery, personalized medicine, and clinical trials.
2. AGI in Education and Personalized Learning
AGI can also be used in education and personalized learning. An AGI machine can personalize learning for each student based on their strengths, weaknesses, and learning style. It can also provide personalized feedback and suggestions for improvement.
3. AGI in Scientific Research and Discovery
AGI can also be used in scientific research and discovery. An AGI machine can analyze large amounts of data, identify patterns, and make predictions. This can lead to new discoveries in fields such as astronomy, biology, and physics.
Conclusion
In conclusion, AGI is the next step in AI. AGI represents a machine that can think and reason like a human and understand language. While AGI is still a long way off, researchers are making significant progress in different areas of AI.
AGI has the potential to transform industries, revolutionize healthcare, personalize learning, and lead to new scientific discoveries.
AI tools to make money and are easy to use📌
— AI Trendz (@AiTrendz) April 18, 2023
1. Build websites 💻 @Durableteam
2. Write Articles ✏ @copy_ai
3. Create Images 🖼 @midjourney
4. Create Music 🎵 @beatovenai
5. Design UI 🌆 @uizard
6. AI Tools ⚒ @AiTrendz #AI #artificialintelligence #aitools #makemoney pic.twitter.com/hhxFxzJUlZ